Search engines only want one thing: To give you the best possible answers to your questions. Only, for a long time, they didn't want you to phrase your question as a question.
The limitations of search engines once made using natural language search difficult. Complex syntax might make it more challenging for your search to return accurate results. Instead of typing “what’s the best recipe for banana muffins,” you throw in the search term “banana muffin recipe.” Your preferred search engine will then spit out a front page of the optimized articles on that subject. It's a state of affairs we're all familiar with.
Now, however, search is catching up. It can handle grammar better and deal with context more effectively. The result? The rise of natural language search.
So, what exactly is natural language search? And how can you optimize your content for it?
In this article, we will explain what natural language search is, why it's the future of SEO, and how you can get on board.
What Is Natural Language Search?
Natural language search is a search request phrased in everyday, conversational language. A straightforward example of a natural language search would be, "How tall is the tallest building in Asia?" You express the search request as you would when talking to another person. More basic examples of natural language search would include:
- "How can I dispose of a mattress?"
- "When was the New York Times founded?"
- "What are the best dogs for families with small children?"
- "How to perform an SEO audit of your website."
Natural language search terms, therefore, fall into the category of "long-tail" keywords. A long-tail keyword is a more extensive, more specific search term. Let's contrast some of our examples above against their short-tail versions:
- "Mattress disposal" vs. "How can I dispose of a mattress?"
- "New York Times founded" vs. "When was the New York Times founded?"
- "Best family dog" vs. "What are the best dogs for families with small children?"
- "SEO audit" vs. "How to perform an SEO audit of your website."
We can already see the potential utility of natural language search. We can express our first two searches just fine as short-tail. Our last two search needs, however, are more specific. It's more likely that you'd find the content you needed more quickly with a natural language search.
As with any other kind of keyword search, you just need to type your natural language search terms into your search bar. You can also use a voice assistant (think Google Home or Siri) to conduct a natural language search. But we'll get to them, and how they're changing the SEO landscape, shortly.
A Short History of Natural language search
"Hold on," you'll say, if you ever used the internet in the '90s, "Wasn't this how search used to work?"
It's true — natural language search isn't a new phenomenon. The early days of the internet saw a lot of natural language use. Ask Jeeves was the most eminent natural language search engine of the '90s. While useful, Ask Jeeves couldn't compete against Google (Ask.com lives on but without Jeeves).
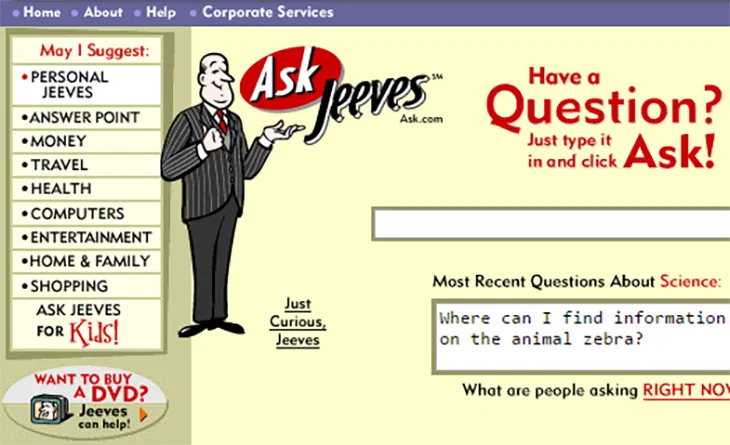
As Google gained prominence as the search engine of choice, keyword searches replaced natural language search. The context and ambiguous intent of conversational phrases confused search engines since they weren't sophisticated enough to make the most of natural language at that point. Keyword-based search helped Google home in on useful results, whereas natural language processing still had a long way to go.
In recent years, however, modern-day search engines have started to catch up.
Introducing BERT
The shift toward natural language search began a few years ago. When Google announced Google BERT, it forecast significant changes for the future of SEO and natural language search.
BERT is a language model used for pre-training search engines according to natural language processing. Google introduced it and made it open-source in 2018. It allows search engines to understand the sensitive context in search requests. Once upon a time, connector words (e.g., “not” or “with”) would overcomplicate the search query. Additional clauses (e.g., “how to rent and insure a car”) would do the same. Now, however, Google can use them to return more sophisticated (and accurate) responses.
Why Natural Language Search Is the Future of SEO
The rise of natural language search comes as part of the overall changes to SEO. As engines gain sophistication, Google drives toward making its results useful and clear. Natural language search is one way, and topical authority is another.
It's not just Google on the march, either. A variety of other engines are optimized to support natural language search. Bing can now also effectively support natural language search.
All these changes help search engines better adapt to changes in search behavior. These new advances in search will help answer more complex search questions.
Increase in Use of Voice Search
The increase of voice search is one reason why natural language has returned to the search engine family. According to Review 42:
- Some 50% of all searches are now voice-activated.
- The same site noted that "72% of people who use voice search devices claim they have become part of their daily routines."
- The same site again noted that "43% of smart speaker owners use the technology to shop."
Naturally, almost all voice searches involve natural language. That's why, from basic informational search requests to ecommerce, natural language search is growing. That represents a vast new search area for SEO pros to target.
More Value in Long-tail
These long-tail keywords have lower traffic, but they also come with less competition and more conversion potential. Long-tail keywords lead to 4.15% more conversions than their shorter cousins. Natural language search will naturally produce more long-tail keywords. And more long-tail keywords mean more potential conversion value for SEO professionals.
More Featured Snippets
Another upshot of BERT is that there are more queries with a featured snippet. Featured snippets are short selections of text that Google posts at the top of searches in an attempt to quickly answer a user’s query. A characteristic example provided by Google concerns the natural language search "parking on a hill with no curb."
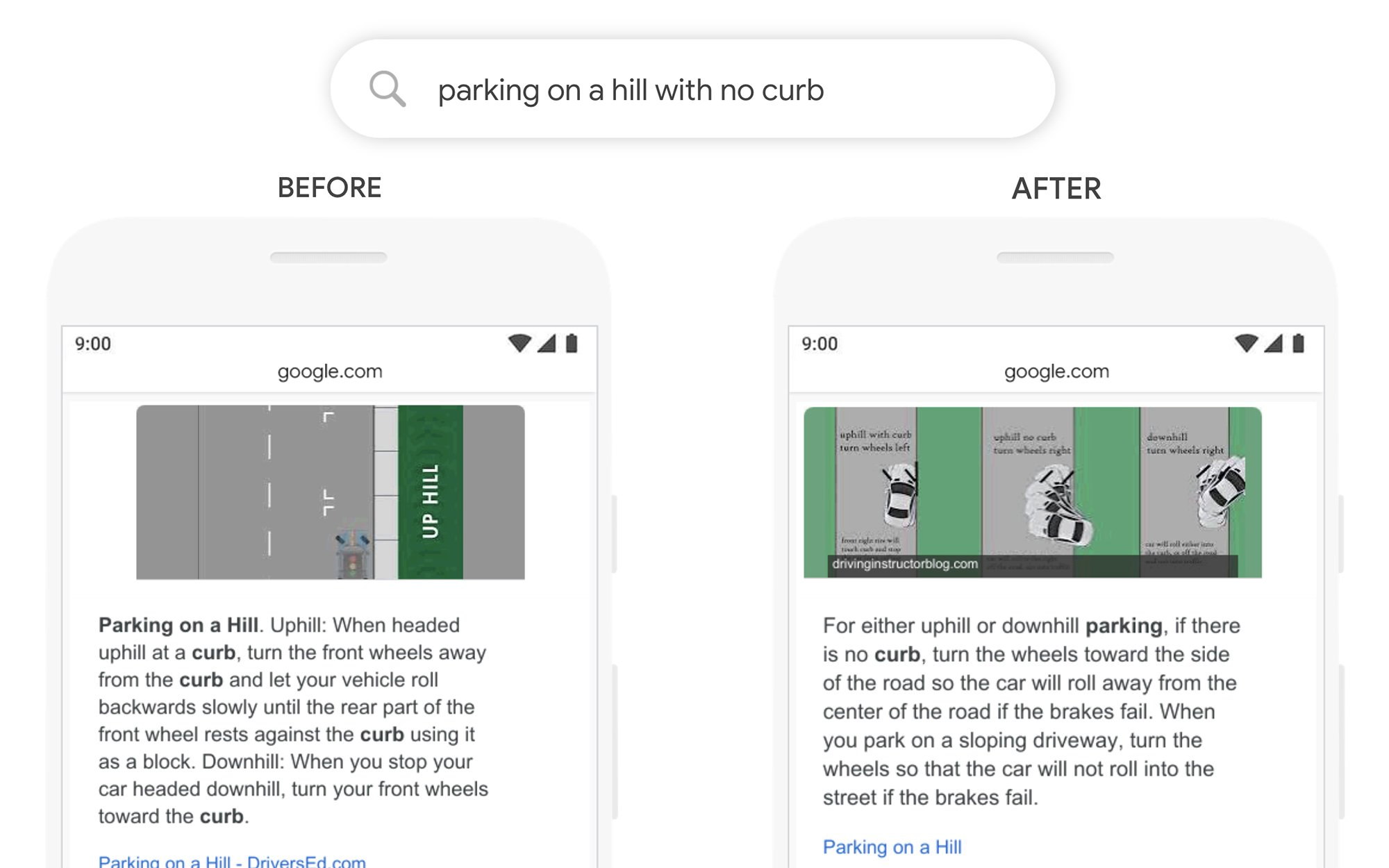
Before BERT, Google's algorithm put too much emphasis on "curb" and not enough on "no." With the added sophistication, Google can return a more relevant result. Given the engine's improved understanding, it also provides more opportunities for SEO professionals to snatch a new featured snippet spot.
Featured snippets are a fundamental part of the SEO pro's arsenal. They become even more critical with the rise in voice search. Google voice search devices often recite featured snippet content when responding to search requests. Thus, BERT and natural language search mean more valuable snippets for SEO professionals to aim for.
How to Optimize Your Site for Natural Language Search
Natural language search opens up new options for SEO pros, so the logical next step is to optimize your site. But doing so isn’t just a case of adding to your keyword stable. You’ll need to take distinct steps to grasp user intent and optimize your posts for quality.
Understand Intent
Intent is vital for search. It's fundamental to Google's new approach to ranking. That goes for topical authority as much as it does for natural language search.
To understand intent, you need to know what questions the searcher is trying to answer. Are they searching to browse? To learn? To buy? To grasp intent, you need to understand why searchers are entering a specific query. Knowing the answers to these questions will give you a sharp sense of how to build content to suit your users’ needs.
Consult your existing user personas when building a picture of intent. Then, use tools like SpyFu's Related Keywords function to see the subjects being spoken about around your primary keyword. You can see the results below for a company specializing in video streaming (where 'video streaming' is the primary keyword).
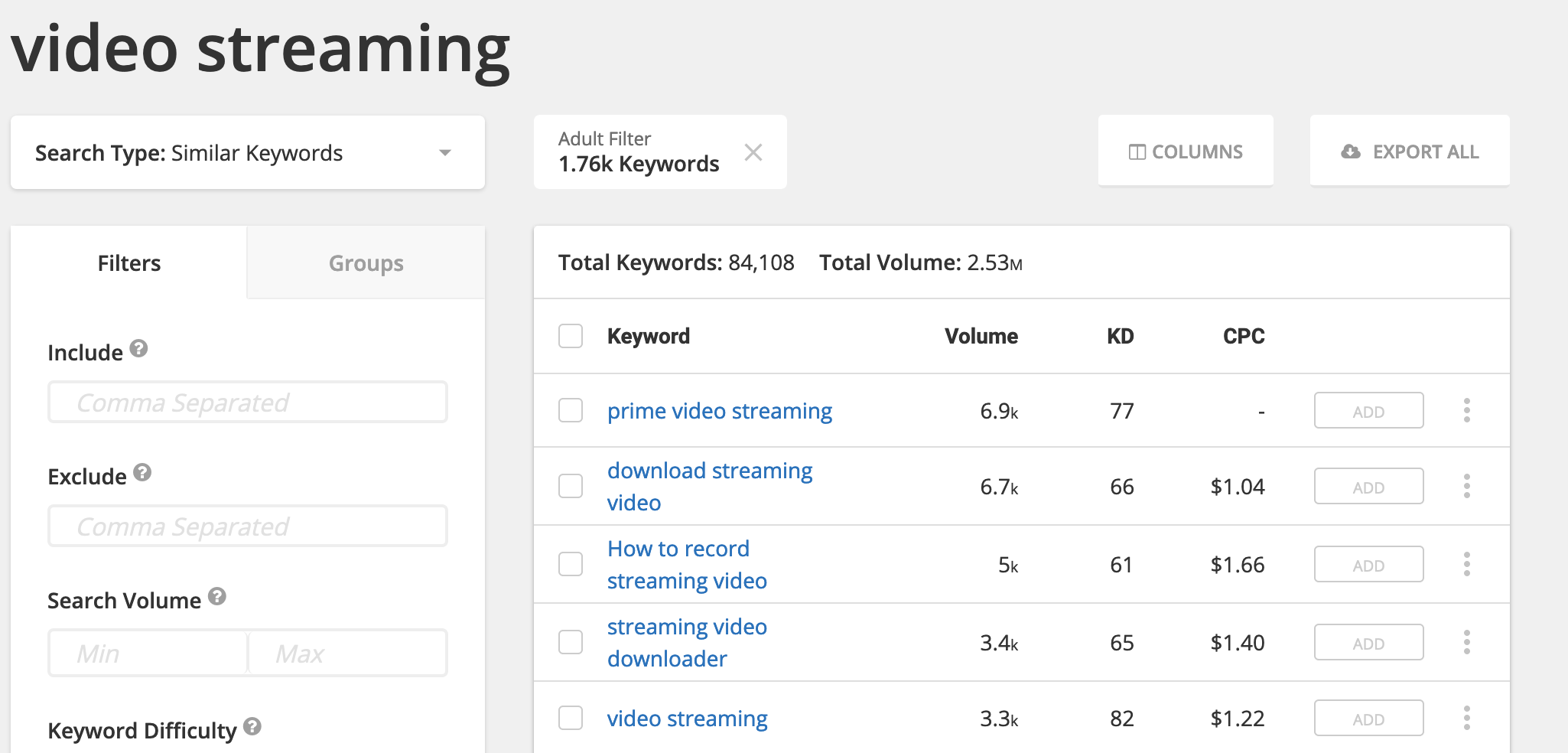
Consider the natural language search term “how to record streaming video.” It has a lower search volume. However, it also has lower keyword difficulty. It would be a good move for the company in question to optimize one or more of their pages for this KW.
Once you've built a keyword list in this way, categorize them into types of intent. Doing so will help you refine your personas. Some searches are transactional, with high conversion potential ("streaming video downloader"). Others are seeking information ("how to record streaming video"). Others will just be seeking a specific address ("prime video streaming").
Now that you have a fuller idea of your personas and their intent, you need to create great content for them. In terms of natural language search, try to anticipate the questions your users are likely to ask. You can also use SpyFu's Related Keywords' Questions function for this.
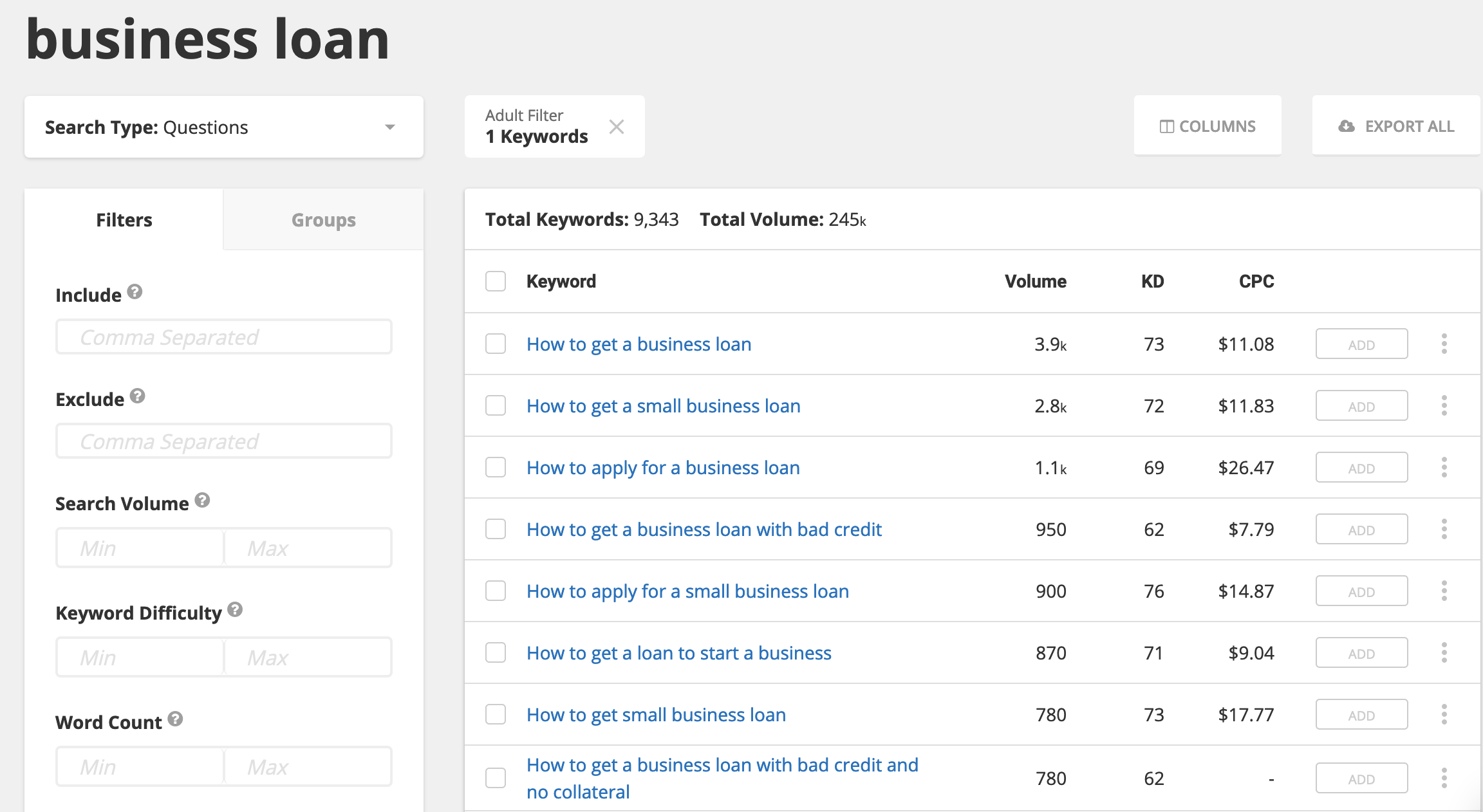
It lists questions related to a given search term and categorizes them by volume, difficulty, and cost-per-click (CPC). Questions are an intrinsic part of natural language search. Predicting the questions is the start. You'll need to create the content to answer the search.
Create informative content
Natural language search is the natural ally of good content. Top industry commentators have noted how "Google BERT and NLP require a strong focus on high-quality, informative content."
After you've determined the questions your users intend on asking, you need to create content that matches that intent.
For example, let's take the natural language search query "how to make poached eggs." After running it through the SpyFu research tool, we can see the organic search ranking analysis for this long-tail keyword. We can then also see that the BBC created the most informative piece of content around it. They make the best of natural language searches for that subject. They're also ahead of the curve for voice-searches for cooking in general.
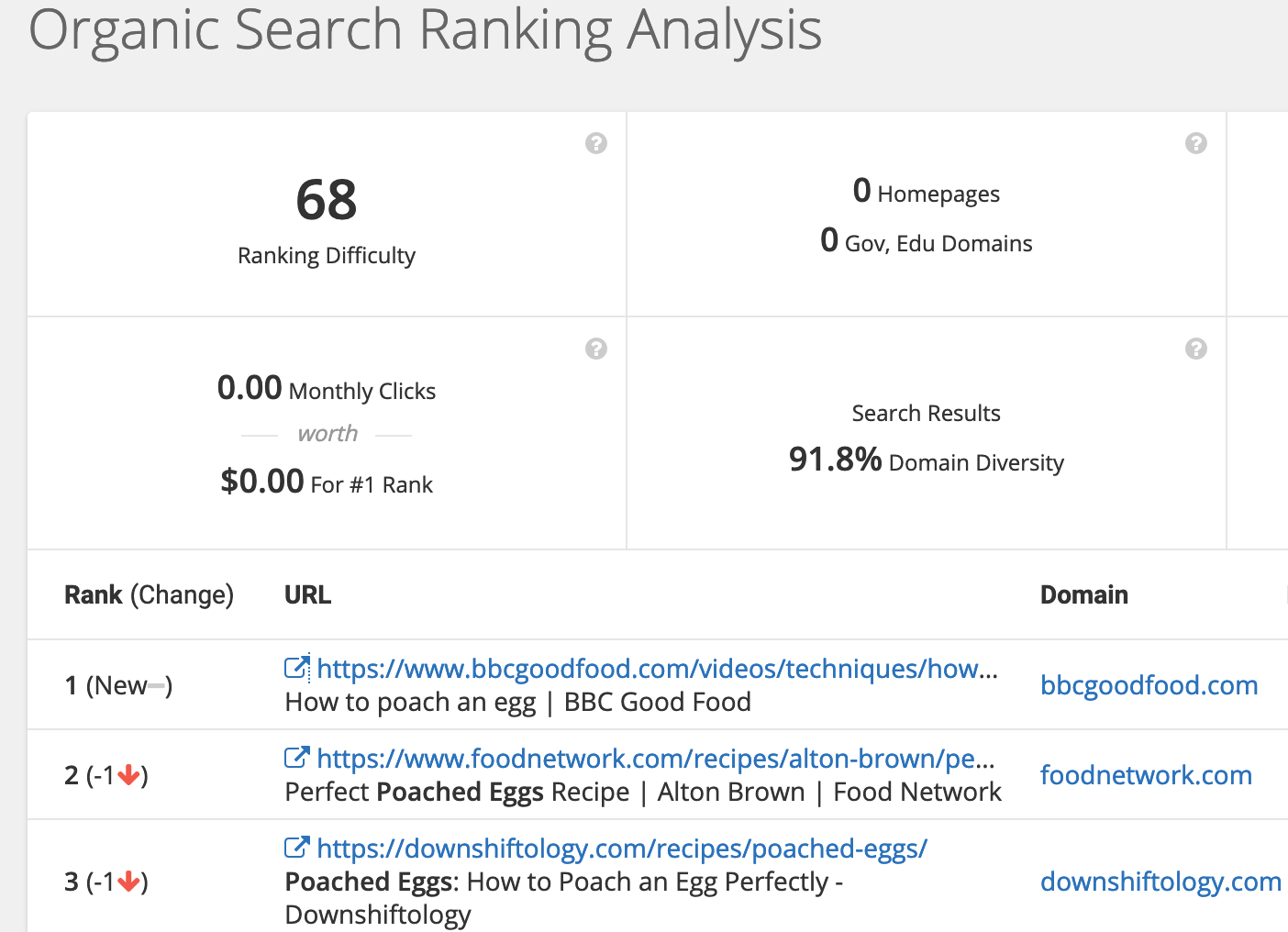
To that end, create content that spells out specific user questions and answers them. For example, let's say SpyFu shows that users frequently ask, "How can I make better investment decisions?" They're likely to ask that question through voice search or another type of natural language search. You might then create an article that uses that question as a title or prominent H2 header. In doing so, you've created content that's well-optimized for natural language search.
If your product has known FAQs, create an article or a series based around them. For example, you can tap an FAQ page, featuring questions like those seen below, for great content:
- Why do I need a CMS?
- How do I get the most out of my CMS?
- How can I integrate my CMS with other products?
- Can I use my CMS for staff training?
- How do I practice good data hygiene with my CMS?
These will be great for capturing high-conversion natural language searches for your product. We suggest turning to resources that might already be in place.
- Ask your support team what questions people ask most.
- Ask your sales team what prospects what to know.
- Search your reviews to see what people aren't sure about when it comes to your site.
- Browse social media to see what people are asking about you.
However you do it, be precise. When optimizing for natural language search, prioritize exact questions. Get specific in your response and elaborate in detail. What you lose in traffic, you can gain in conversions.
...But Don’t Forget Related Keywords
As you develop content, be sure to include related keywords for two reasons.
- Your reader might have wondered about a similar phrase that you can address.
- It helps to expand your reach on similar searches.
Again, the right tools will make your search easier. Below, you can see similar keywords from a SpyFu search for "how to get a loan." Several of the keywords and questions included would make great H2 headers in an article about loan applications. A savvy content manager might build them out into hub-and-spoke content. Doing so will help optimize the article and site for these natural language search terms.
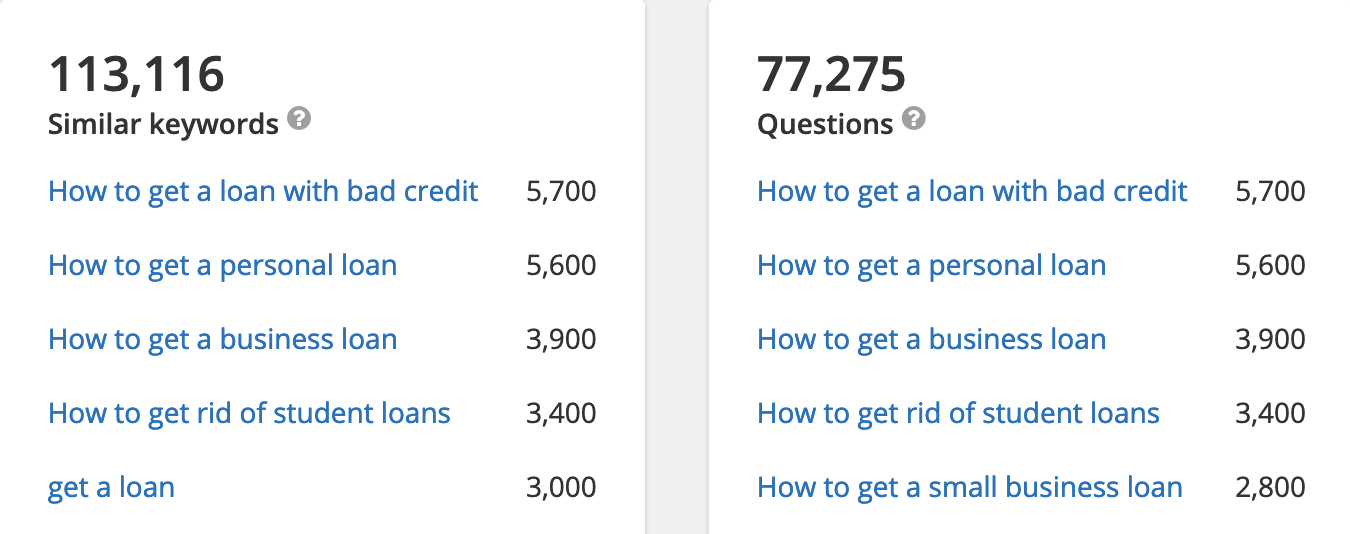
Use your Tech Stack
Post-BERT natural language search relies on semantic search. For that reason, it's a great time for SEOs who want to get the best out of natural language search to use Schema Markup.
Schema is a kind of semantic vocabulary that helps Google understand information on a page better. It allows you to tag content on your page relative to its itemscope (e.g., “[company name]”) and itemtype (e.g., “Fintech startup”). Companies that use Schema will get better SERP returns. Furthermore, natural language searches are more likely to find sites that are well-optimized for Schema. You can learn more about implementing Schema here.
There are other non-SEO tools that can help you optimize your site for natural language search. For example, business-to-business SEOs looking to optimize for their buyer personas' natural language search questions might try CallRail or Chorus.ai. Both solutions offer Conversation Intelligence, which highlights essential phrases in call transcripts. If multiple customers ask the same questions on calls, other potential customers will likely ask the same, and you can leverage these as natural language search terms.
Remember, Google wants to provide people with the best answers to their questions. Your best bet for ranking is to anticipate those questions and answer them thoroughly.
A More Natural Future
Natural language search and other kinds of search associated with it are here to stay. Not only that — they're paying out, too. For instance, Juniper Research predicts that voice-search-driven commerce alone will bring in $80 billion per year worldwide by 2023.
For an SEO, anticipating precisely what your buyer personas will ask can be tricky. Even still, making a site natural-language friendly is vital. Those companies that embrace it can look forward to significant advantages across their content marketing.